NPTEL Deep Learning Week 9 Assignment Answers 2025
1. What can be a possible consequence of choosing a very small learning rate?
a. Slow convergence
b. Overshooting minima
c. Oscillations around the minima
d. All of the above
Answer :- For Answers Click Here
2. The following is the equation of update vector for momentum optimizer. Which of the following is true for y?
V, = yVe-1 + nVeJ(0)
a. y is the momentum term which indicates acceleration
b. y is the step size
c. y is the first order moment
d. y is the second order moment
Answer :-
3. Which of the following is true about momentum optimizer?
a. It helps accelerating Stochastic Gradient Descent in right direction
b. It helps prevent unwanted oscillations
c. It helps to know the direction of the next step with knowledge of the previous step
d. All of the above
Answer :-
4.
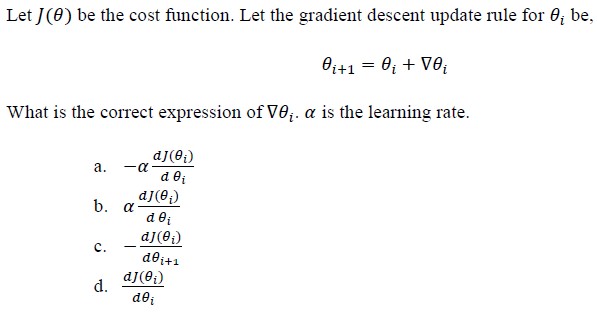
Answer :-
5. A given cost function is of the form J(0) =6 02 – 60+6? What is the weight update rule for
gradient descent optimization at step t+1? Consider, a to be the learning rate.
a. 0t+1 = 0t – 6a (20 – 1)
b. 0t+1 = 0t + 6a (20)
c. 0t+1 = 0t – a(120 – 6 + 6)
d. 0t+1 = 0t – 6a(20 + 1)
Answer :-
6. If the first few iterations of gradient descent cause the function f(0o,01) to increase rather than
decrease, then what could be the most likely cause for this?
a. we have set the learning rate to too large a value
b. we have set the learning rate to zero
c. we have set the learning rate to a very small value
d. learning rate is gradually decreased by a constant value after every epoch
Answer :- For Answers Click Here
7. For a function f(0o,01), if 0o and 01 are initialized at a global minimum, then what should be the
values of 0o and 01 after a single iteration of gradient descent?
a. 0o and 01 will update as per gradient descent rule
b. 0o and 01 will remain same
c. Depends on the values of 0o and 01
d. Depends on the learning rate
Answer :-
8. What can be one of the practical problems of exploding gradient?
a. Too large update of weight values leading to unstable network
b. Too small update of weight values inhibiting the network to learn
c. Too large update of weight values leading to faster convergence
d. Too small update of weight values leading to slower convergence
Answer :-
9. What are the steps for using a gradient descent al gorithm?
- Calculate error between the actual value and the predicted value
- Update the weights and biases using gradient descent formula
- Pass an input through the network and get values from output layer
- Initialize weights and biases of the network with random values
- Calculate gradient value corresponding to each weight and bias
a. 1, 2, 3, 4, 5
b. 5, 4, 3, 2, 1
c. 3, 2, 1, 5, 4
d. 4, 3, 1, 5, 2
Answer :-
10. You run gradient descent for 15 iterations with learning rate n = 0.3 and compute error after
each iteration. You find that the value of error decreases very slowly. Based on this, which of the
following conclusions seems most plausible?
a. Rather than using the current value of a, use a larger value of n
b. Rather than using the current value of a, use a smaller value of n
c. Keep n = 0.3
d. None of the above
Answer :- For Answers Click Here